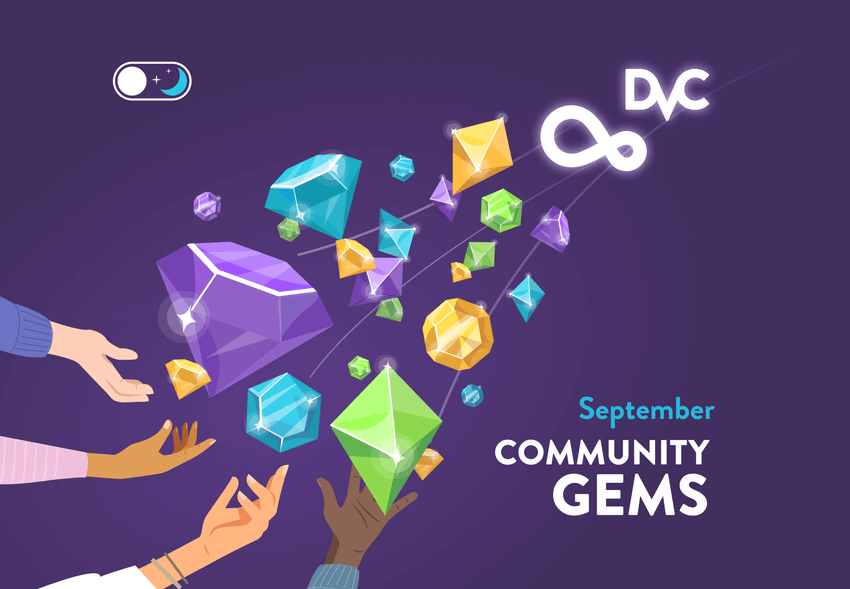
Is there a way to share data across multiple on-premise machines so that users can train models individually?
This is a good scenario to try out one of these use cases:
You can have a single storage location mounted on each workstation to serve as a central cache.
That way all of your machine learning engineers can work with the same data in a central location.
Thanks for the question @fchpriani!
If we change the remote we are using in our workspace, does that effect where DVC pulls and pushes data to for all historical commits?
Thanks for bringing this up @mattlbeck!
Right now DVC just uses whichever remote is configured in a respective commit that you've checked out.
To clarify things a bit more, if you run dvc push/pull
in a workspace with a
new remote, that new remote will be used for --all-branches
, --all-tags
, and
--all-commits
.
Is there a command to execute only a few specific stages in a DVC pipeline?
You can freeze the stages that you do not want to be executed.
dvc freeze
and dvc unfreeze
help you do this. Or you can use
dvc repro --glob pattern*
together with -s
to match the stages you want to
run.
Thanks for the question @LucZ!
When running queued experiments, is it expected for DVC to run dvc checkout
for each experiment?
This brings up a good point, so thanks @dmh!
If you usually run experiments with dvc repro
, you'll notice that it doesn't
checkout any files. That's because the experiment is running in the current
workspace.
When you use dvc exp run --queue
or dvc exp run --run-all
, it runs each
experiment in its own separate temp workspace, so files have to be checked out
into those workspaces. Check out the notes in
this reference doc on queueing and parallel execution
for more details.
When working with a data registry, is it possible to pull a specific project folder, modify it, then push Git changes and dvc push
to the remote storage without pulling data from all the directories?
This is definitely possible. The most common way to handle this is by working in
the specific folder. You can dvc pull -R
from the sub-directory, then make
your changes in the sub-directory, and dvc add
the changes. Then you can do a
git commit
and dvc push
to manage those changes.
You can also use a Git sub-repo and a DVC sub-repo to do this if each folder has
a distinct project. Use git init
and dvc init
in the project folders and
then you can pull them down, modify, commit and push commit back.
Really good question @ross.tsenov!
Is it possible to auto-generate reports with metrics and plots by running DVC in a CML job when the data is stored in AWS bucket instead of GitHub?
Thanks for asking @Masmoudi!
When you need to retrieve data, you can run dvc pull
to get it from the S3
bucket. If you run into an error with this, try adding
uses: iterative/setup-dvc@v1
to the dvc pull
command. This could happen
because the default CML action doesn't install DVC.
If you want more details on how CML works in GitHub, check out the docs!
What mechanism can I use in GitLab to trigger a CI pipeline periodically so that models get re-trained and logged to DVC automatically?
You can use
pipeline schedules to
train your model periodically and dvc push
the results.
Good question @mihaj!
At our October Office Hours Meetup we will be going over how to get started with data version control. RSVP for the Meetup here to stay up to date with specifics as we get closer to the event!
Join us in Discord to get all your DVC and CML questions answered!