As GenAI Fever Fades - Time to Prioritize Robust Engineering Over Overblown Promises
The data stack generation is determined by a blend of emerging technologies and the applications they enable. We are now entering a new phase of data stack development, characterized by the adoption of foundational models, generative processes, faster time-to-value, and reduced data demands.
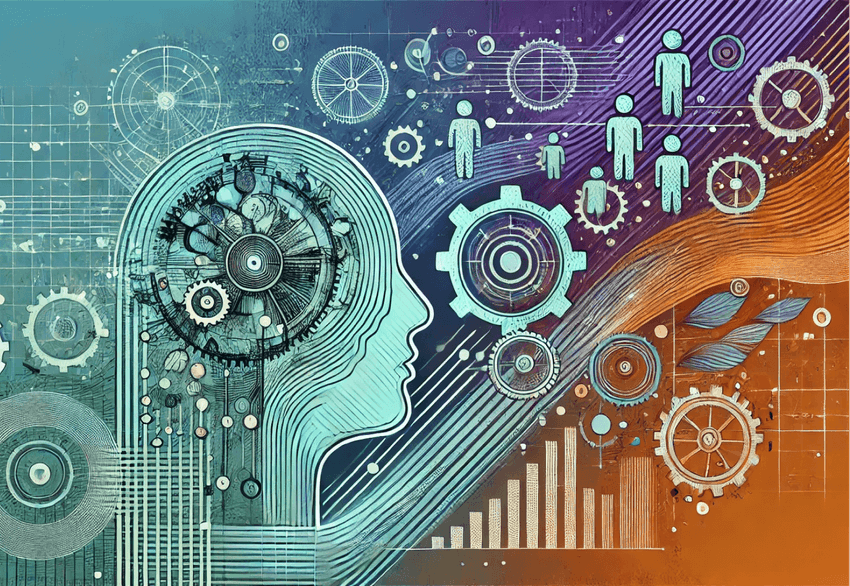
Image generated by DALL-E
Artificial Intelligence (AI) and Machine Learning (ML) existed long before OpenAI announced ChatGPT on November 30, 2022, but it was on that day that the hype began in earnest.
Less than two years after GenAI was introduced on a global scale, the company reported that 92% of Fortune 500 companies are using OpenAI’s technologies. Everywhere you turn, an IT vendor is announcing some form of generative AI assistant or workflow. While the potential for AI innovation is incredible and very real, the AI bloom seems to be coming off the rose.
One symptom is the lack of AI in production environments today. We’re still treating AI as a shiny new toy that’s not ready for prime time. Why? Because it is not ready for prime time!
As the AI “bubble” begins to deflate, the market actually benefits in several significant ways. Historically, we have witnessed similar patterns with other technologies, where initial excitement around groundbreaking innovations moves into what Gartner calls the trough of disillusionment and eventually to a more mature, engineering-focused approach (i.e., dot-com, cloud). This transition is crucial for the sustainable growth and practical application of AI. Watch as the market moves away from purely speculative GenAI applications and towards practical, engineering-driven solutions.
In the early stages of technological advancements, excitement often surrounds new models and breakthroughs. For instance, when AlexNet's deep convolutional neural network (CNN) architecture emerged in 2012, they were hailed as revolutionary, offering a novel way to handle data and aiming to solve the whole computer vision problem. These early phases are marked by rapid experimentation and exuberance, where the focus is on exploring the potential of new technologies. Sound familiar?
However, as these technologies mature, the focus inevitably shifts from excitement to engineering. This evolution reflects a broader trend in technology development, where initial breakthroughs are followed by a period of rigorous refinement and practical implementation. The same is now happening with AI. The current phase involves transitioning from the initial excitement of advanced LLM models to a more structured approach emphasizing engineering, production, and data curation.
This shift is beneficial for several reasons. During the initial excitement, models can sometimes produce unpredictable results, as seen with early chatbots that generate unexpected outcomes (Did you hear about the Chevrolet dealer whose ChatGPT was “tricked” into selling a 2024 Chevy Tahoe for $1?). By focusing on engineering, we can develop more stable systems that deliver consistent value. We've had the Beta, and now we need 1.0 (and beyond).
This maturation process (with a focus on engineering) helps address critical data quality and scalability issues. The need for high-quality, pre-processed data becomes increasingly important as AI technologies become more integrated into everyday applications. Engineering solutions focusing on data pre-processing, validation, and management will facilitate more accurate and reliable AI systems. This, in turn, will enhance the overall effectiveness of AI in practical applications, from business solutions to consumer products.
Beyond tackling data quality and scalability concerns, this necessary shift of focus towards engineering innovation will lead to the development of tools and frameworks that better support AI workflows, including handling large volumes of unstructured data (including images and videos). That, in turn, will foster a more collaborative and integrated approach between AI and data management practices. As the AI and data stacks evolve to complement each other, we can expect more cohesive and innovative solutions that address AI implementation's technical and operational challenges. This alignment will facilitate smoother integration of AI technologies into existing systems and workflows, ultimately driving more widespread adoption and utilization.
Looking ahead, this maturation process promises substantial benefits beyond the realm of developers and engineers. Just as the dot-com bubble burst led to the refinement and widespread adoption of internet technologies, the current focus on data pre-processing and engineering in AI will pave the way for transformative applications across various industries. Imagine AI-powered healthcare diagnostics that rely on meticulously curated data sets or financial systems that leverage AI for predictive analytics to manage risks more effectively. These advancements aren't just about enhancing technical capabilities; they're about improving outcomes for society as a whole.
While the leak in the AI bubble may seem like a setback, it represents an important step toward the industry's maturity and stability. The AI sector can overcome early challenges by focusing on engineering, data management, and practical implementation and delivering more reliable, valuable, and impactful solutions—the result – more robust tools and frameworks that will benefit engineers and data scientists.
What are your thoughts on this topic? Join the conversation!